Research
Our group engages in researches about condensed matter theory and material science engineering. Some of our research topics are listed below:
Floquet Engineering on Quantum Materials
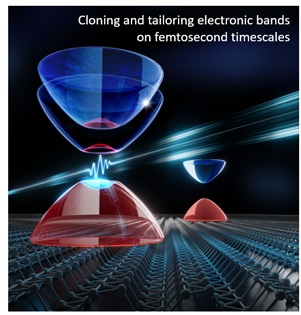
Time-periodic fields provide unprecedented opportunities for tailoring the quantum states of matter by Floquet engineering. In solids, the periodic arrangement of atoms leads to electronic structure, which is periodic in momentum. In analogy, time-periodic drive can lead to Floquet states, which are periodic in energy. More importantly, the interaction between electrons in the material and time-periodic driving field can further lead to modifications of the electronic structure, symmetry, and topological properties, etc. Such Floquet engineering can result in light-induced emergent phenomena that are otherwise not possible in the equilibrium, for example, turning a topologically trivial material into a topological nontrivial material, realizing topological superconductivity far away from equilibrium, etc.
References:
- Physical Review B 111, L081106 (2025).
- npj Quantum Materials 9, 101 (2024).
- Physical Review Letters 131, 116401 (2023).
- Electronic Structure 5, 2 (2023).
- Nature 614, 75-80 (2023).(News & Views: Nature, Phys.org, Tsinghua.)
- Nature Reviews Physics 4, 33–48 (2022). (Editors’ Suggestion)
Ultrafast Dynamic Phenomena in Quantum Materials
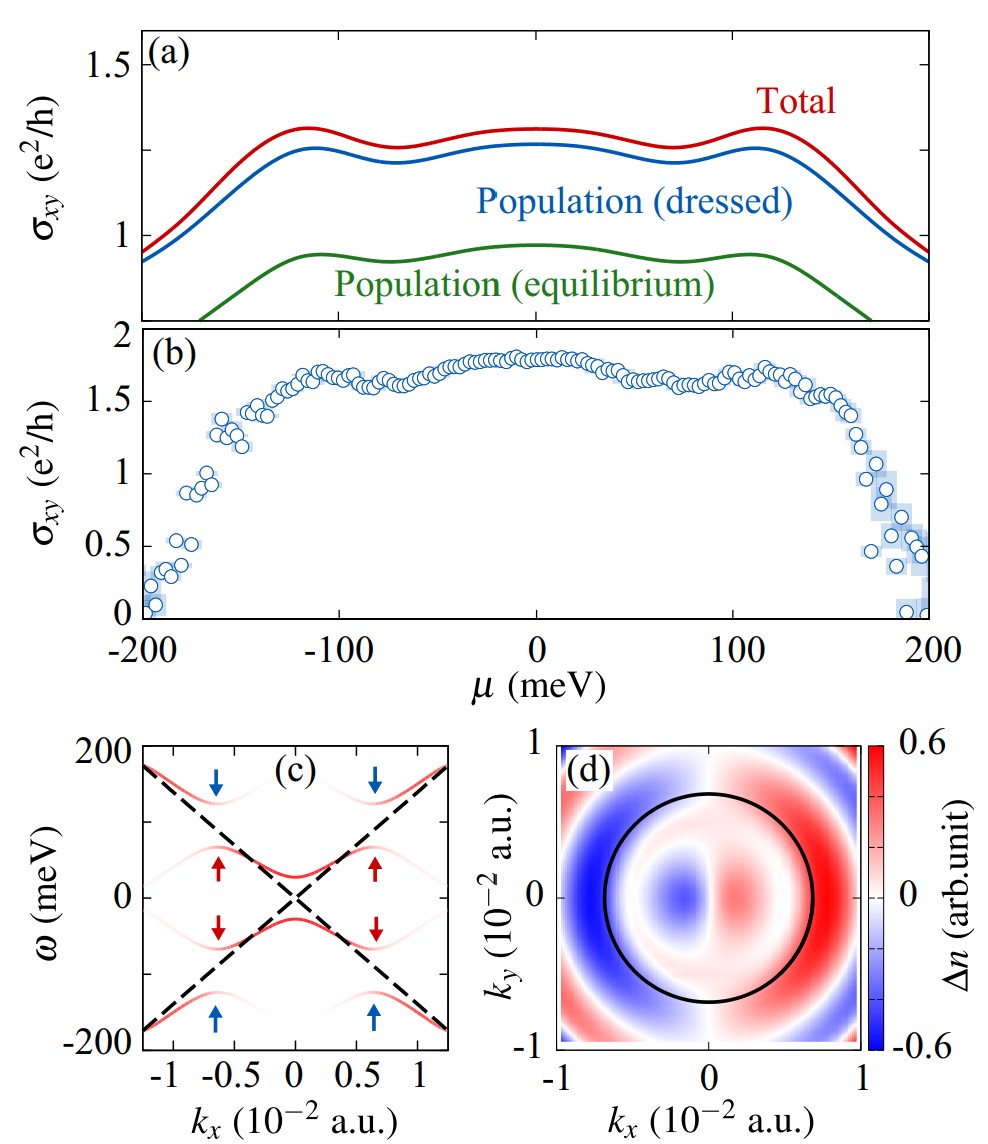
Optical driving is a good way to excite new state of matter and tune the properties of materials in non-equilibrium. Based on the TDDFT and master equations, we explore the transport properties of massless and massive Dirac fermion systems under the photon fields. We argue the non-trivial Hall current have both contributions from optical field driven Berry curvature and charge imbalances.
References:
- Nature 632, 273–279 (2024).
- Nano Letters 24 (22), 6592–6600 (2024).
- Nature Communications 15, 4406 (2024).
- Nano Letters 24 (21), 6278–6285 (2024).
- Physical Review Letters 132, 016603 (2024). (Editors’ Suggestion)
- Nano Letters 23 (22), 10282-10289 (2023).
- Nature Materials 21, 773–778 (2022). (News & Views: Nat. Mater.)
- New Journal of Physics 21, 093005 (2019).
- Physical Review B 99, 214302 (2019).
Topological Magnetic Materials
The Dancing between topological materials and magnetism will bring new concepts and phenomena to exotic materials, which are key for the new applications in spintronic devices. Based on theoretical tools (DFT and kp theory), our group mainly focus on the following topics.
· AFM topological systems
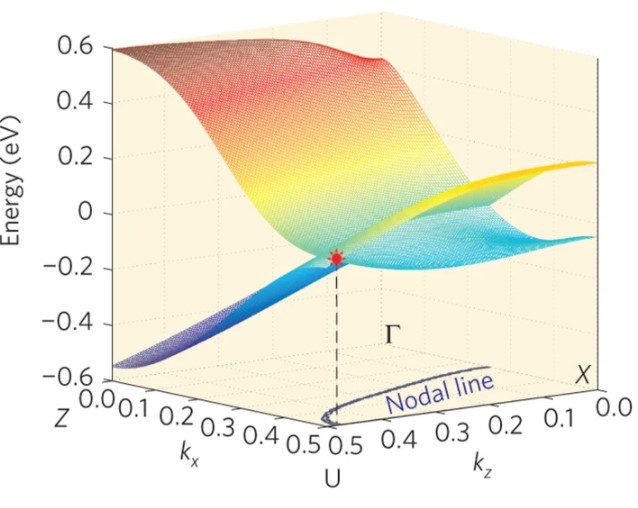
Antiferromagnetic topological materials have showed great possibilities in not only research of the interplay of Dirac fermion physics and magnetism, but new-generation low-power memory design. We predicted AFM topological fermions in CuMnAs
References:
- Physical Review Research 2 (2), 022025 (2020).
- Physical Review B 96, 224405 (2017).
- Nature Physics 12, 1100 (2016).
· Topological Material Engineering
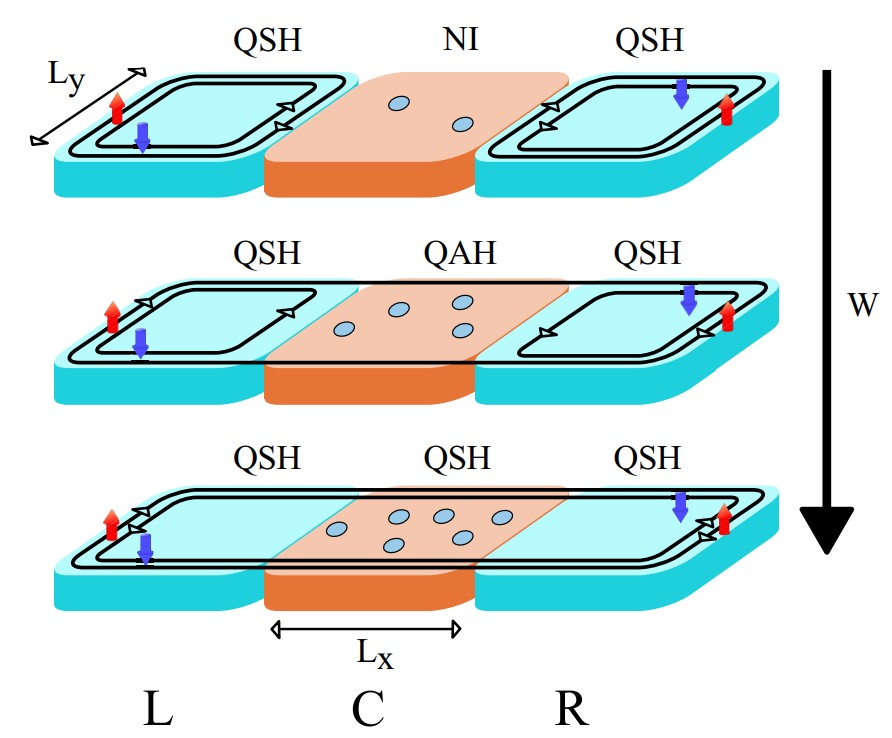
Due to the strong SOC in topological materials, the degrees of freedom of spin and momentum could couple together. Via using the symmetry arguments, we design ways to engineer magnetic and topological properties.
References:
- Physical Review B 102, 201405 (2020).
- Physical Review B 98, 245108 (2018).
- Physical Review Letters 115, 136801 (2015).
- Nano Letters 15, 2031 (2015).
- Science 339, 1582 (2013).
- Physical Review Letters 111, 116601 (2013).
· New Topological Materials
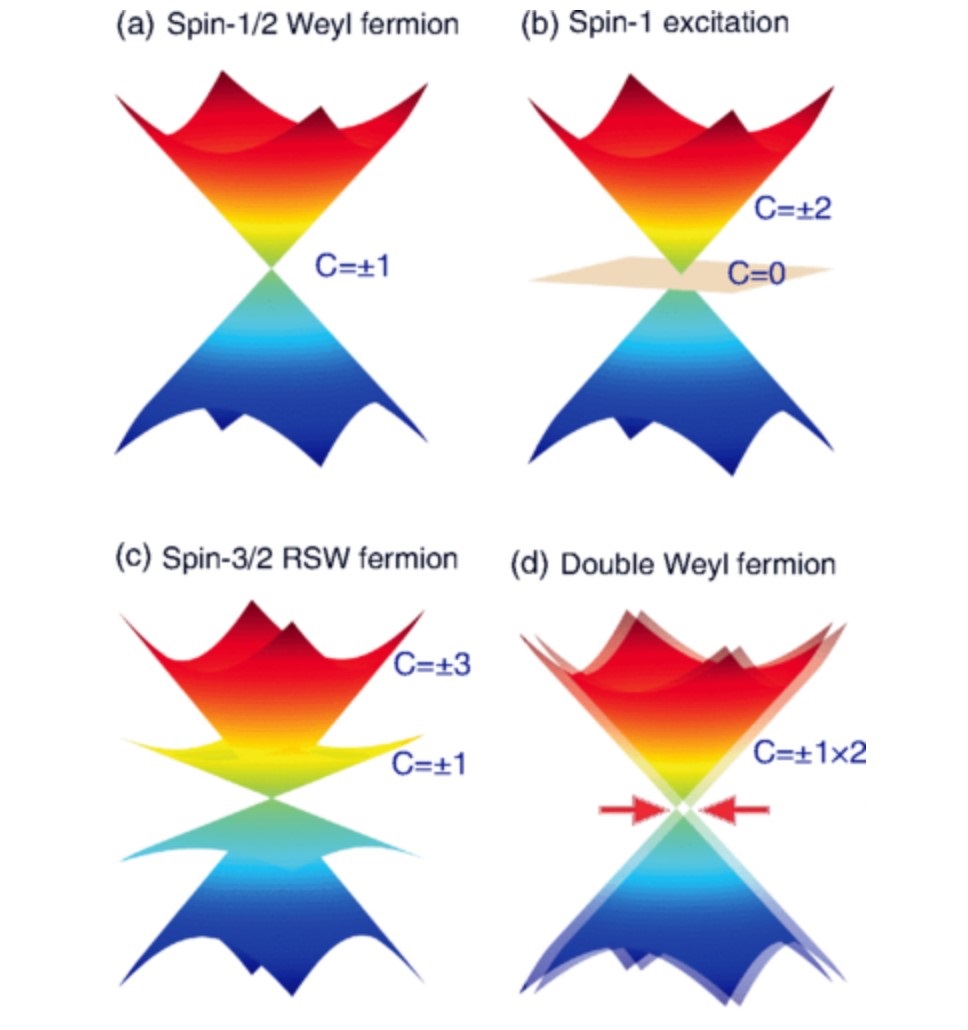
We predicted the new fermions in CoSi and many new topological materials, including 2D quantum spin Hall insulator in dumbbell stanene, the Dirac fermion in hexagonal ABC compound LiZnBi, weak TI in BiTeI-Bi2-BiTeI sandwiched structure and so on. Those topological materials are equipped with many exotic quantum properties and provide material candidates for novel physics and future applications.
References:
- Physical Review Letters 119, 206402 (2017).
- Physical Review B 96, 115203 (2017).
- Physical Review B 93, 241117(R) (2016).
- Physical Review B (R) 90, 121408 (2014).
- Physical Review B 89, 041409(R) (2014).
- Physical Review Letters 112, 056801 (2014).
- Physical Review Letters 111, 136804 (2013).
Machine Learning for Material Sciences
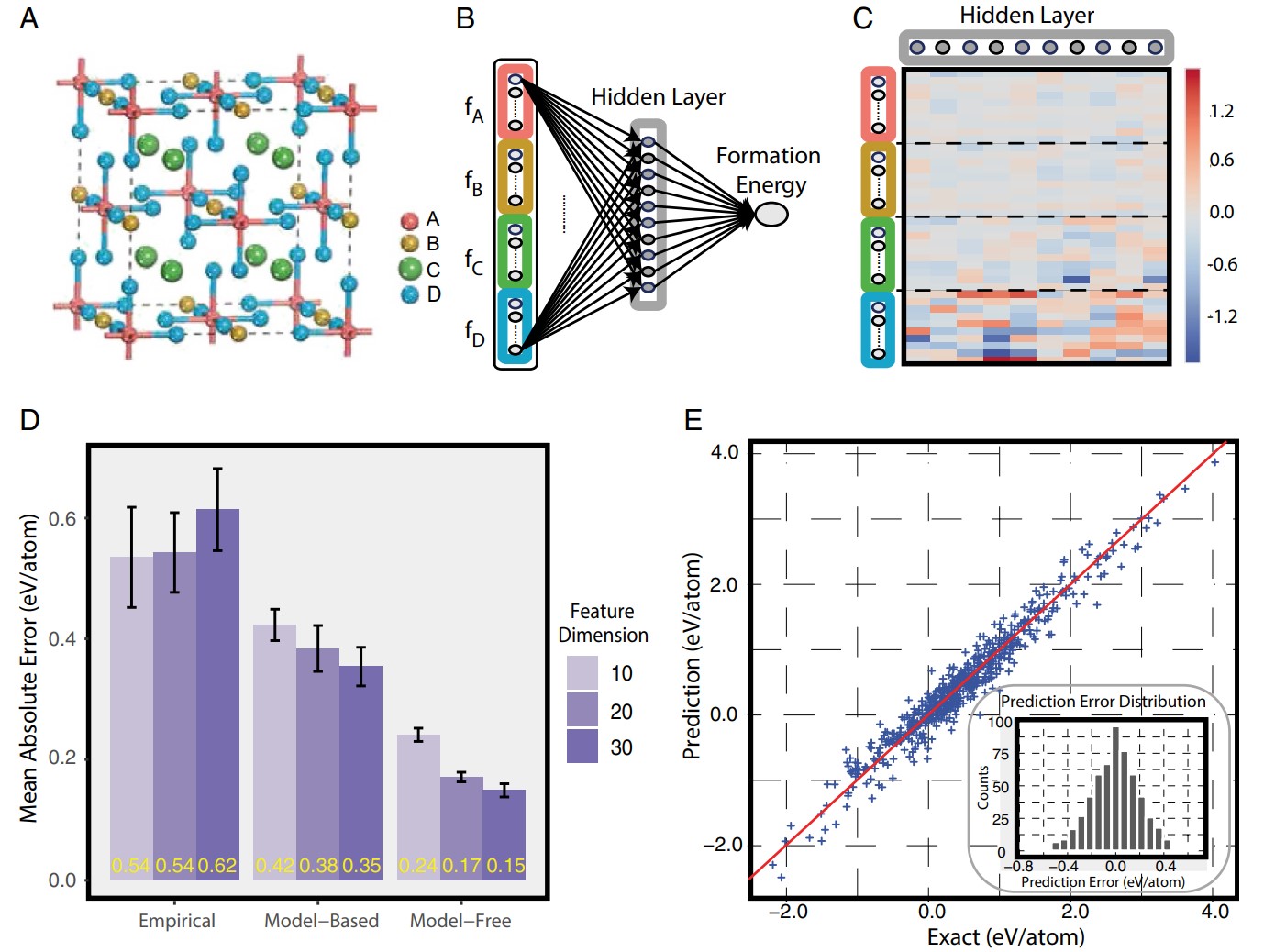
Exciting advances have been made in artificial intelligence (AI) during recent decades. Among them, machine learning and deep learning techniques are widely used in almost all parts of science. Our group engages in applying machine learning to material science, makes full use of neural networks and other machine learning models, and expects to find machines to learn the basic properties of atoms by themselves from the extensive database of known compounds and materials.
References: